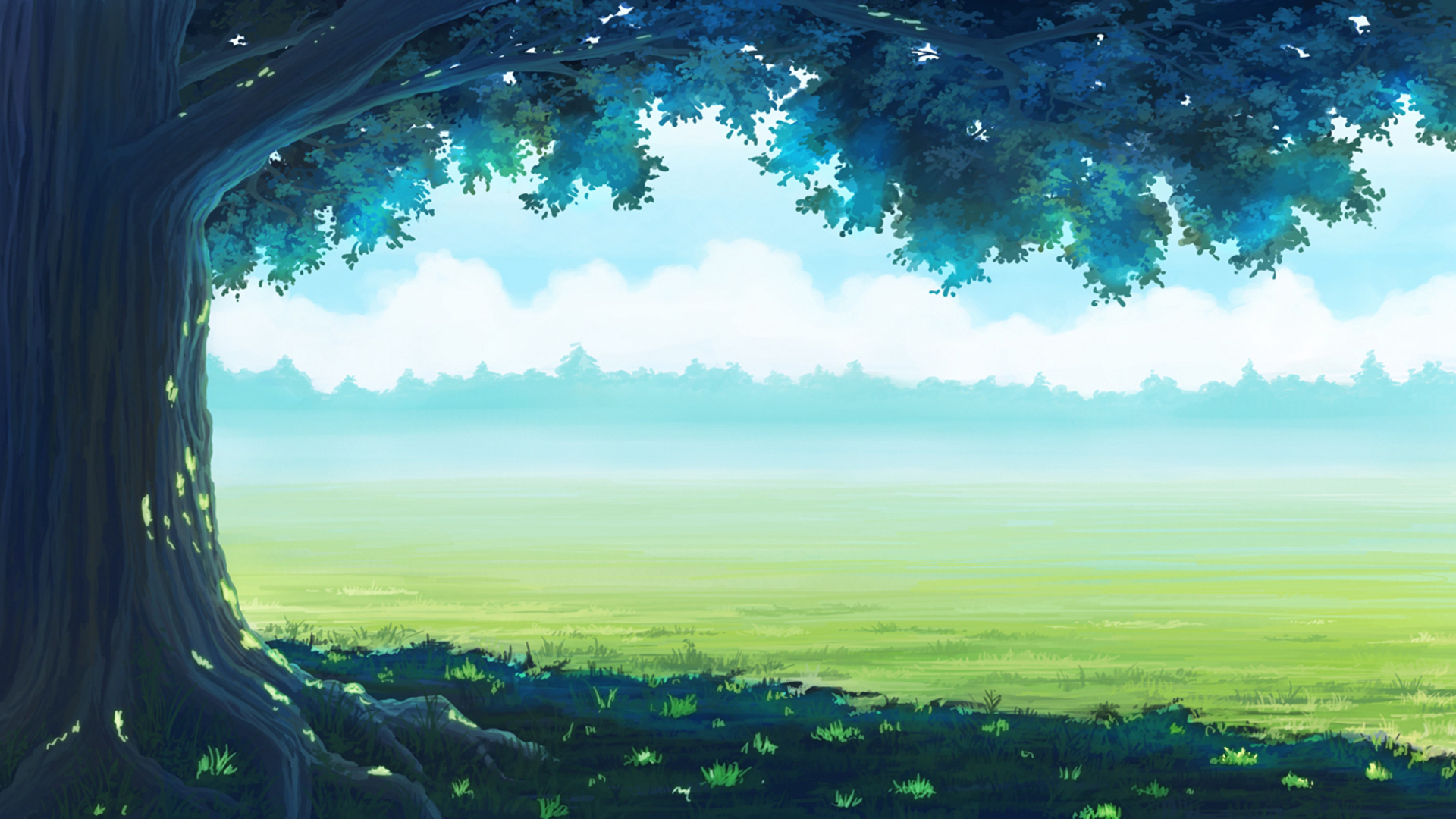
Tensorflow2.1-note
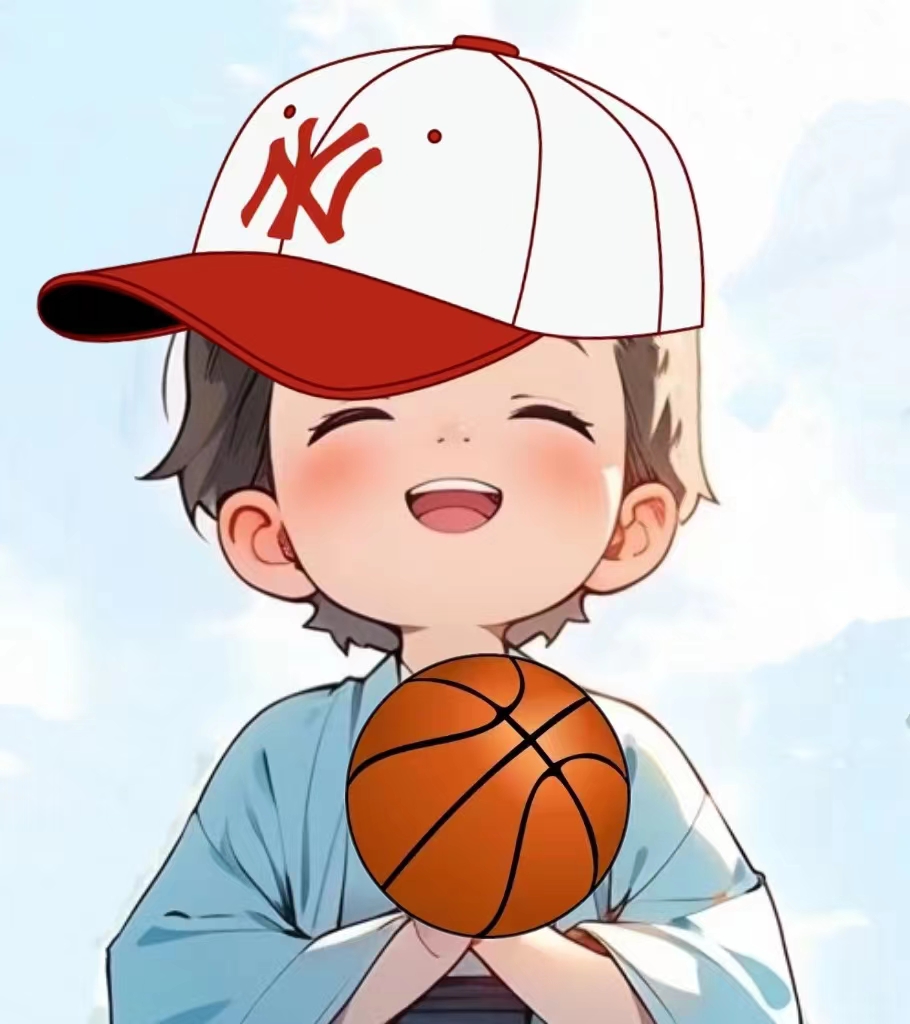
基本知识
张量
为什么要在TF2中使用张量?
tensorflow中的数据类型
- np.array
- tf.constant()
- tf.Variable() 将变量标记为“可训练”
1 | array = np.array([1,2,3,4]) |
结论:np.array和tf.constant没有区别?
张量的表示方法
张量的维数判定
等号后面有几个[,就是几维张量。
1 | scalar = 1 |
维度的表示
一维 | 二维 | 多维 | |
---|---|---|---|
维度表示 | 元素个数 | [行数,列数] | [n,m,j,k……] |
axis的含义
对于张量x, shape = (n,m,j,k)
,对应的axis就是(axis=0,axis=1,axis=2,axis=3)
张量的索引
1 | x[m,n,p] # 从最外部的方框逐渐缩小到固定元素 |
张量的创建:
指定内容 tf.constant / numpy转化 tf. convert_to_tensor / 特殊结构 tf.zeros等
1 | # tf.constant(张量内容,dtype=数据类型(可选)) |
张量的操作函数
1 | # 计算张量维度上元素的最小值 tf.reduce_min(张量名, axis=操作轴)) |
指定axis的含义:该axis上的元素作为查找目标/取平均值对象,遍历其他axis上的所有值,最终结果的结构是去除该axis后的值。比如a
的shape是(2,3,4)
,对axis = 1
运算,最终结果的shape就是(2,4)
1 | # 同理有reduce_mean / reduce_sum |
数学运算
对应元素的四则运算:tf.add,tf.subtract,tf.multiply,tf.divide
平方、次方与开方:tf.square,tf.pow,tf.sqrt
矩阵乘:tf.matmul
常用操作
梯度计算
with结构记录计算过程,gradient求出张量的梯度
1 | # with tf.GradientTape( ) as tape: |
梯度计算的注意事项
-
参与梯度计算的数据类型需要是float
-
计算梯度的时候可以直接写
+-*/
,但不能写^
-
二阶导数的计算
1
2
3
4
5with tf.GradientTape() as tape2:
with tf.GradientTape() as tape1:
y = a*tf.pow(x,2) + b*x + c
dy_dx = tape1.gradient(y,x)
dy2_dx2 = tape2.gradient(dy_dx,x) -
多元函数的导数怎么表示
1
2
3
4
5
6with tf.GradientTape() as tape:
x1 = tf.Variable(tf.constant(3.0))
x2 = tf.Variable(tf.constant(3.0))
y = tf.pow(x1,2)*x2
grad = tape.gradient(y,[x1,x2])
print(grad) -
偏导怎么计算?
常用函数
tf.one_hot(待转换数据, depth=几分类)
1 | classes = 3 |
data = tf.data.Dataset.from_tensor_slices((输入特征, 标签)) 切分传入张量的第一维度,生成输入特征/标签对,构建数据集
1 | train_db = tf.data.Dataset.from_tensor_slices((x_train, y_train)).batch(32) |
自减函数 assign_sub
1 | w.assign_sub(1) |
NN复杂度
空间复杂度
总参数= 总w + 总b
时间复杂度
乘加运算次数(计算线的个数)
模块处理
基本流程
1 | import |
数据导入
控制batch
- 在
model.fit
中设置batch_size - 底层控制
训练
学习率设置
指数衰减学习率 = 初始学习率* 学习率衰减率(当前轮数/ 多少轮衰减一次)
正则化缓解过拟合
loss = loss( y与y_ )+ REGULARIZER * loss(w)
模型的保存与载入
自动进行参数的保存与载入
1 | checkpoint_save_path = "./checkpoint/mnist.ckpt" |
手动进行参数的保存和载入
1 | # 保存权重 |
训练结果的保存
通过history = model.fit
记录训练结果
1 | # history=model.fit(训练集数据, 训练集标签,batch_size=, epochs=, validation_split=用作测试数据的比例,validation_data=测试集, validation_freq=测试频率) |
history.history
内部也就只有这4个list
实例程序
TF底层实现
1 | # 利用鸢尾花数据集,实现前向传播、反向传播,可视化loss曲线 |
Keras
1 | import tensorflow as tf |
- Title: Tensorflow2.1-note
- Author: Charles
- Created at : 2023-03-10 15:54:03
- Updated at : 2023-07-27 16:45:50
- Link: https://charles2530.github.io/2023/03/10/tensorflow2-1-note/
- License: This work is licensed under CC BY-NC-SA 4.0.