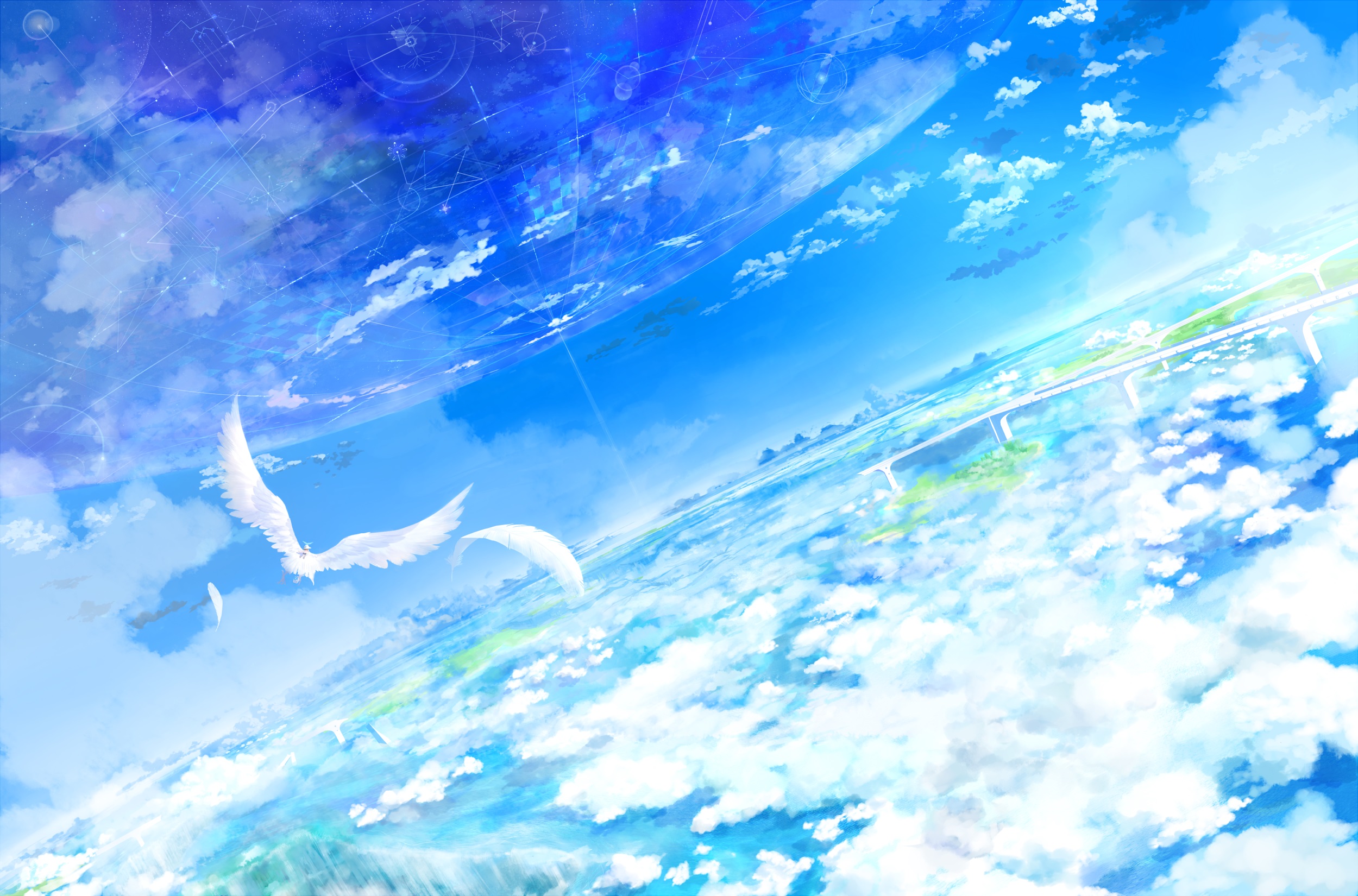
TOPSIS-model
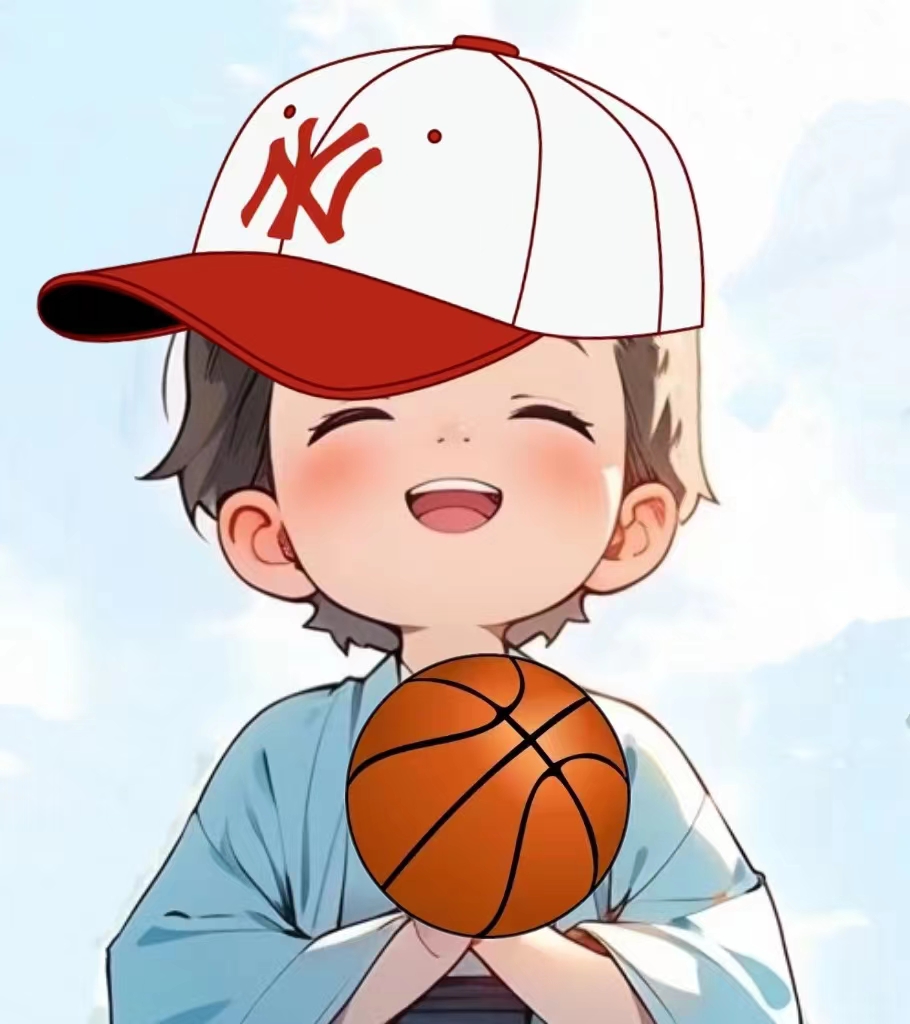
TOPSIS模型基础
TOPSIS基本概念与建模
TOPSIS的概念
TOPSIS (Technique for Order Preference by Similarity to an Ideal Solution )模型中文叫做“逼近理想解排序方法”,是根据评价对象与理想化目标的接近程度进行排序的方法,是一种距离综合评价方法。
TOPSIS建模
TOPSIS建模基本思路是通过假定正、负理想解,测算各样本与正、负理想解的距离,得到其与理想方案的相对贴近度(即距离正理想解越近同时距离负理想解越远),进行各评价对象的优劣排序。
TOPSIS模型数据预处理
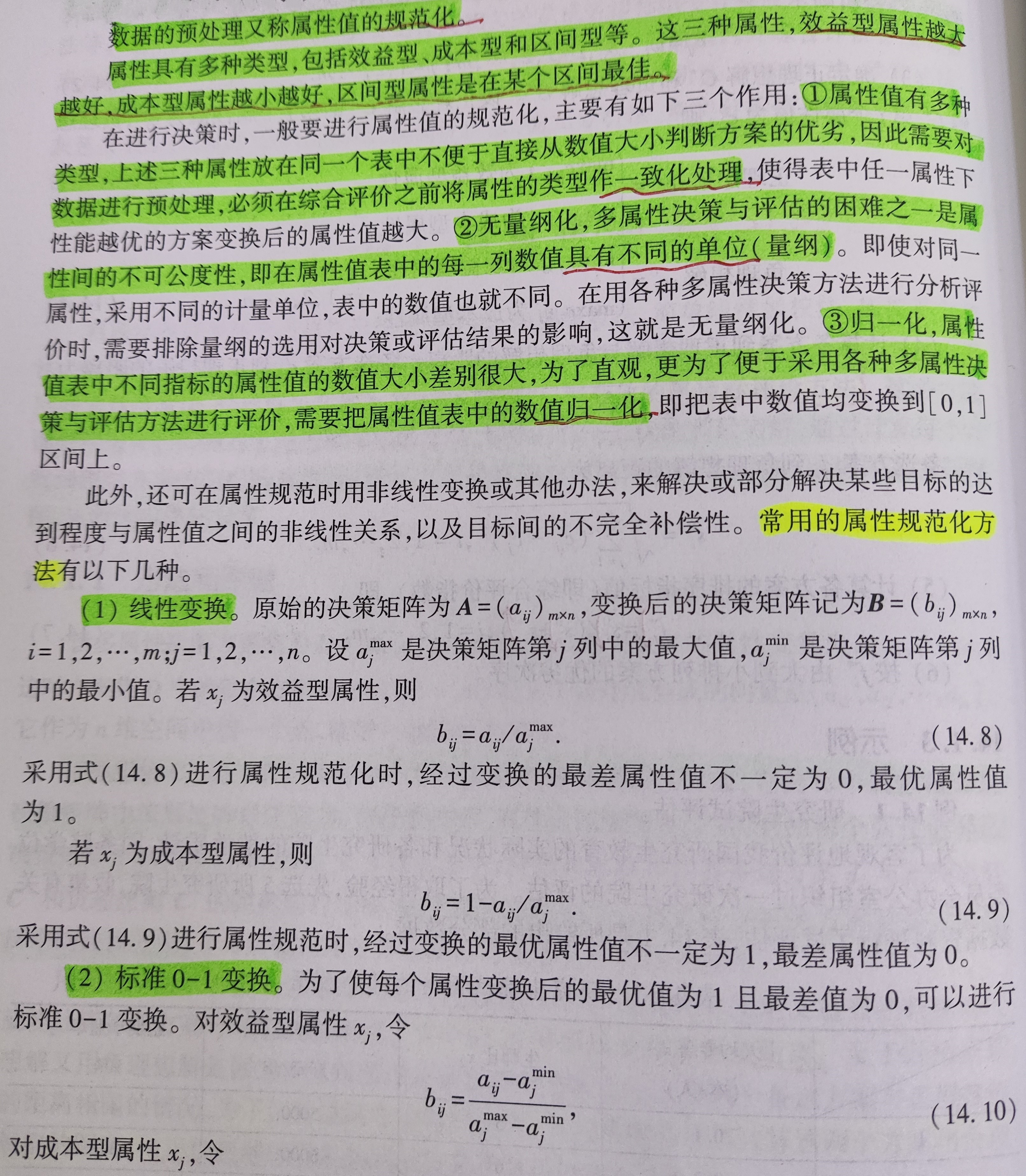
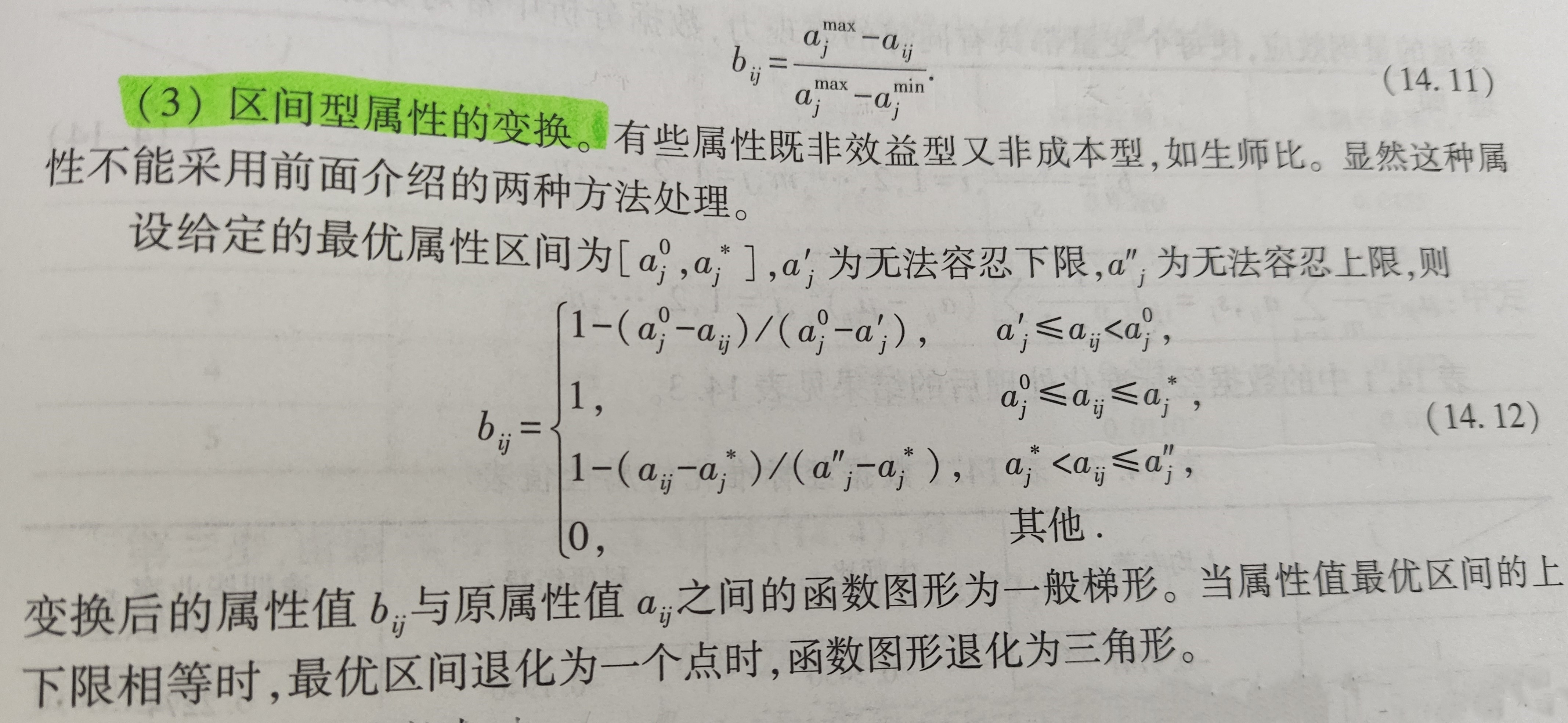
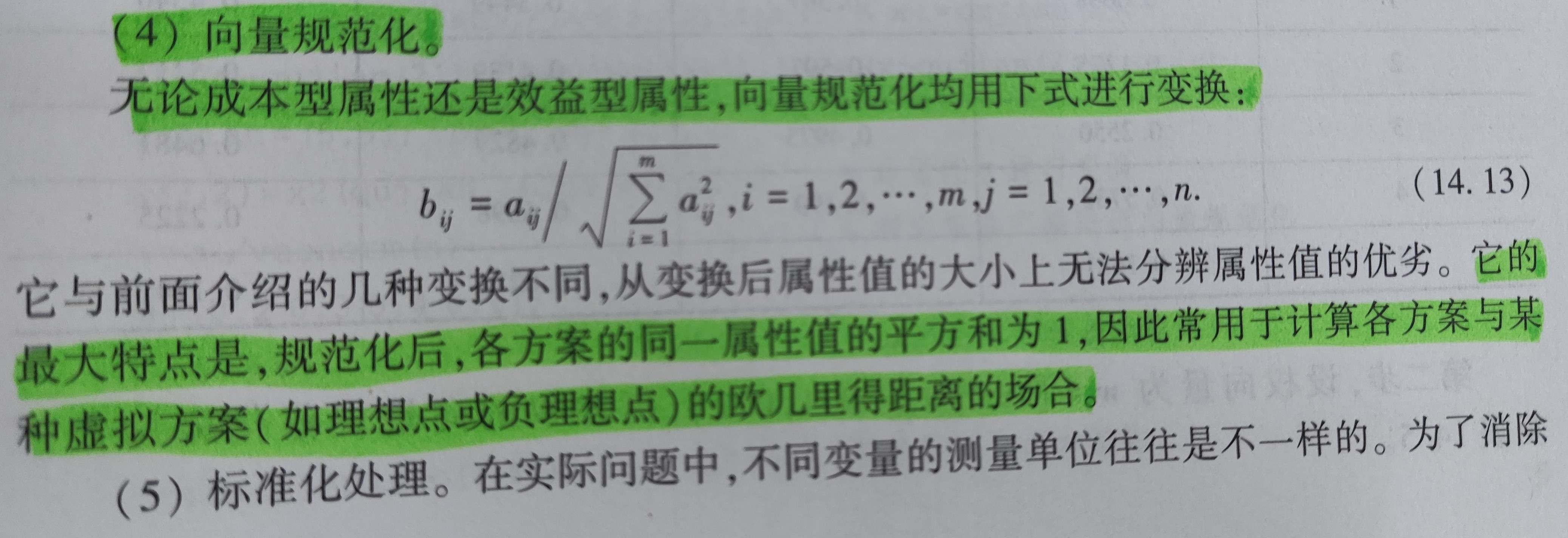
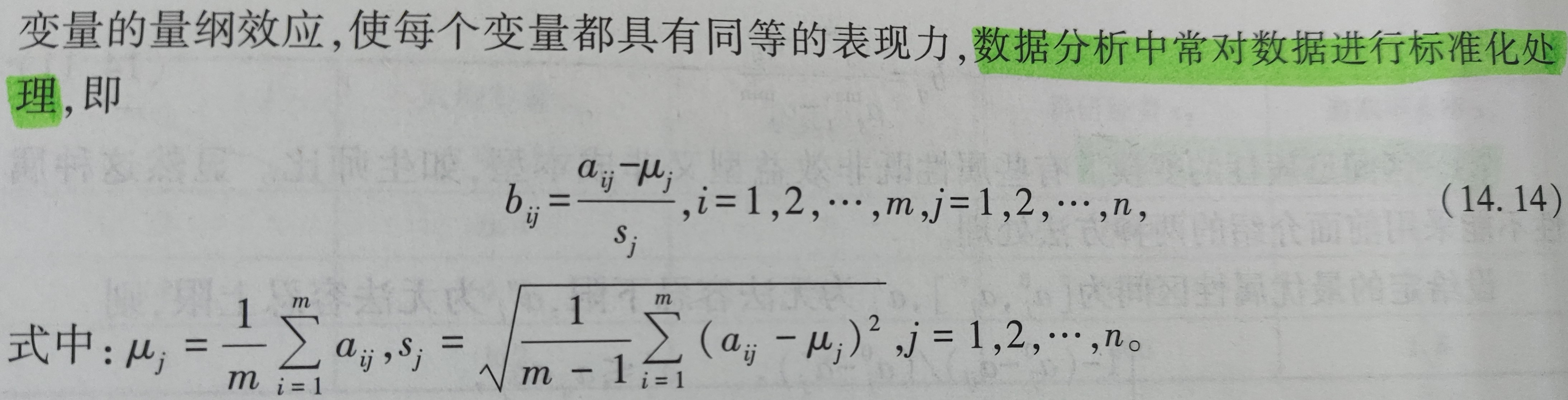
TOPSIS法的算法步骤
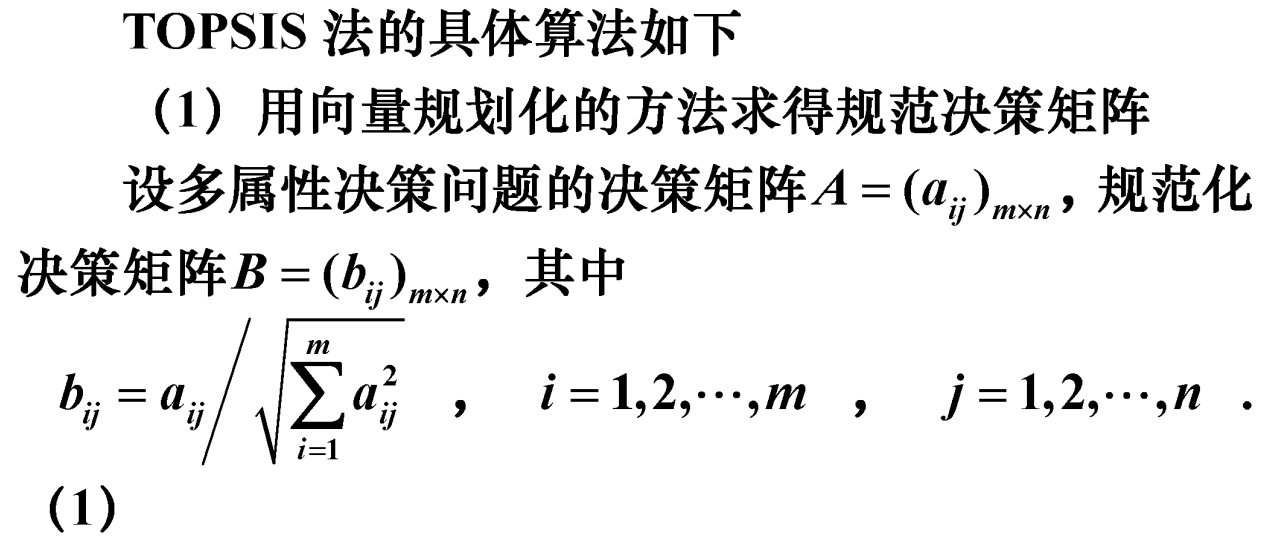
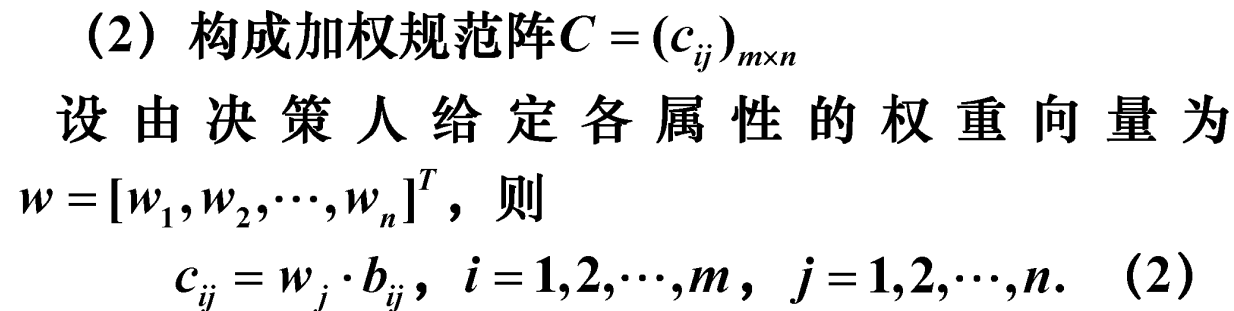
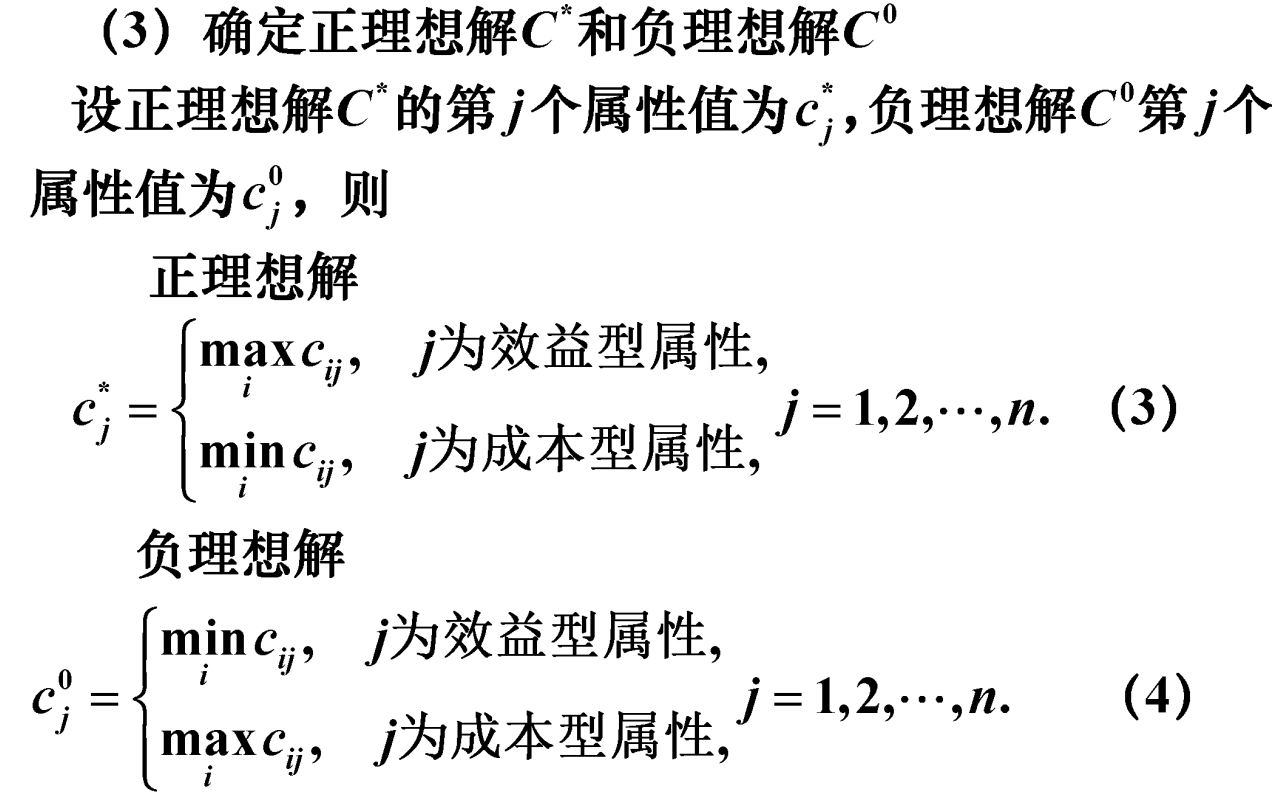
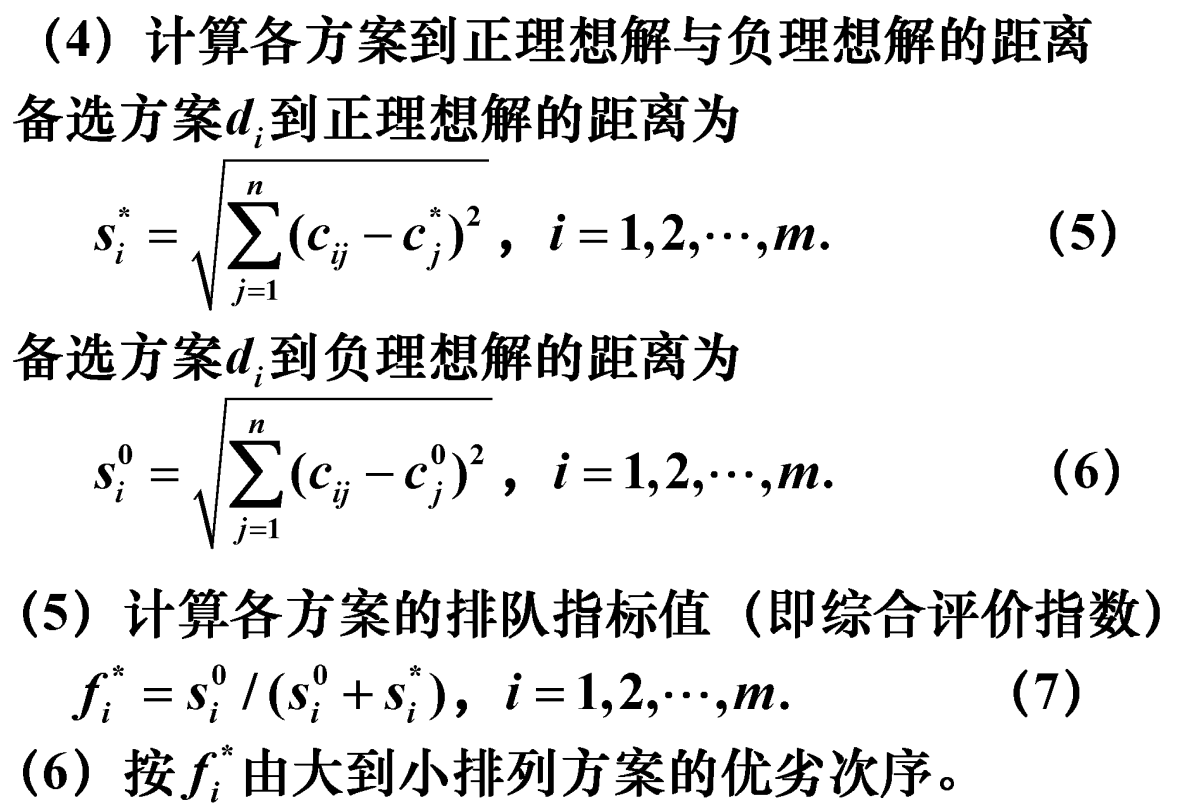
模型适用范围
TOPSIS模型为处理评价类问题的模型,适用于指标相对较多且各指标之间相对独立的情况下使用,简而言之,需要大量数据。
MATLAB实现示例
1 | %Topsis算法基本思想:基于归一化后的原始数据矩阵,采用余弦法找出有限方案中的最优方案和最劣方案(分别用最优向量和最劣向量表示), |
- Title: TOPSIS-model
- Author: Charles
- Created at : 2023-09-01 22:48:56
- Updated at : 2023-09-04 15:24:05
- Link: https://charles2530.github.io/2023/09/01/topsis-model/
- License: This work is licensed under CC BY-NC-SA 4.0.
recommend_articles
recommend_articles
Comments